Pearson cuts borrowings by £100m using AI-based cash forecasting
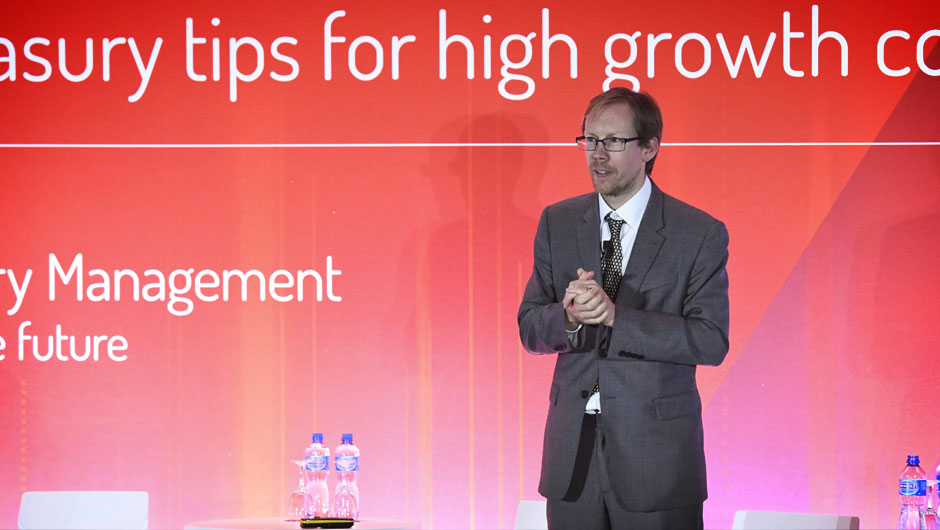
By deploying an AI-supported forecasting solution, education company Pearson has improved processes, reduced float by over £100m and minimised unexpected costs.
Treasurers everywhere struggle to forecast future cash flows effectively, with common challenges ranging from manual treasury processes to insufficient visibility over cash balances.
And companies pay a significant penalty by having to hold excess cash or use borrowing facilities in order to cover unexpected cash requirements.
But now, new technologies like AI and RPA are bringing new opportunities in forecasting. One company to take the plunge into next-generation forecasting is education firm Pearson, as James Kelly, SVP, Group Treasurer at Pearson, outlined in a presentation at the recent EuroFinance Strategic International Treasury conference in Miami.
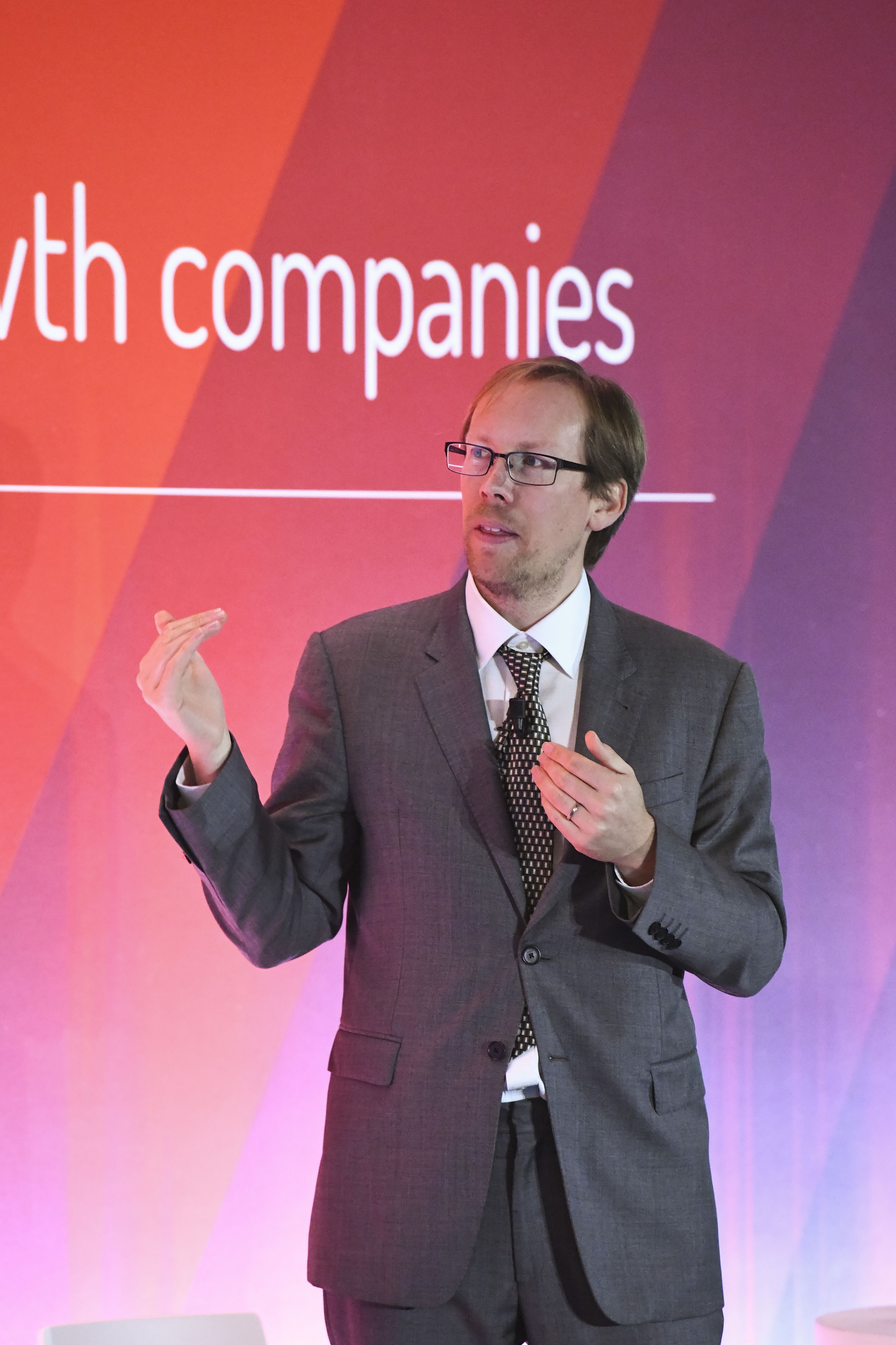
Moving target
Accurate cash forecasting is an important issue for Pearson, not least because the education company’s cash cycle is highly seasonal. “During the first six months of the year we have significant outflows in the hundreds of millions of dollars as we invest ahead of ‘back to school’ and we pay royalties and dividends,” Kelly explained. “This then swings round so that we generate heavily in the second half of the year.”
However, until recently the company’s TMS-based forecasting process had been heavily reliant on manual input and the judgement and experience of local finance teams. This naturally led to some inconsistency in the approach taken by different teams when preparing forecasts.
With Pearson’s business model increasingly moving online – of the company’s £4.1 billion turnover in 2018, only a third came from non-digital business – Kelly was also keen to observe the impact of this shift on working capital and the cash cycle. “I wanted to be able to look at the business, understand it and start to be able to add value and test hypotheses,” he said. To compound the challenge, the company is currently in the process of shifting some of its finance organisation into shared service centres, meaning that placing such heavy reliance on local teams was no longer sustainable.
Against this backdrop, Kelly was looking for a forecasting system that would be both highly effective and relatively low impact for finance staff. The existing TMS-based process “wasn’t an awful lot better than Excel”, and tracking the variance between forecasts and actuals was a challenge. Further, Kelly discovered that “a lot of the insights we were bringing in terms of our commercial partnership were based on gut feel rather than hard data.”
Harnessing AI
In adopting a new solution, Kelly wanted to gain the ability to drill down by operating company or by process in order to drive behavioural change. This began with a forensic exercise to understand the group’s data sources and to classify cash flows by type to make analysis easier. Process improvements were also needed to improve predictability. As Kelly commented, “If you haven’t got predictability, you can end up overriding your forecast and saying ‘nine days out of ten I’m spot on, but there’s the risk that one day out of ten I’ll be miles out’ – so you decide to hold a lot of cash back just in case.”
The company opted for Cashforce, attracted by its product’s ability to integrate with the group’s systems, its use of robotics to avoid the need for manual keying and its multiple AI algorithms offering greater insights into cash flow.
With Pearson in the process of moving to a single ERP system, the company had plenty of access to sales data, which provided information on expected customer receipts in the coming 30 days. “But if I want to do a three-month forecast, I’m going to have to do something that gets me beyond the month,” Kelly noted. “That’s where the AI kicks in and starts to build patterns based on pre-existing trends.”
Getting up and running
With IT already heavily focused on digitising the business, Kelly was keen to ensure that the workload for IT was kept low. Compliance and procurement processes were intensive – but once the contracts were agreed, a functional prototype was built within a month, and subsequently scaled up. The process of developing the forecasts is now a partnership of the treasury team and the system, using insights from local teams. “The more rules and insights you can provide, the better the output will be, so we’ve done a lot of work to really understand the drivers of our cash flows,” said Kelly.
Since then, the company has already seen significant results. For one thing, more accurate forecasting has enabled Pearson to reduce its peak borrowing requirements substantially. During 2018, Pearson’s revolving credit facility (RCF) drawings ranged from undrawn to a peak of £411m, according to recent financial reports. Kelly said that the use of AI-based forecasting had reduced overseas balances over £100m – a reduction of around 50% of the non ‘trapped’ cash balance which were used to fund its seasonal working capital requirements in place of RCF drawings.
Without this increase in available cash, peak borrowings would have been at least £100m higher and the company’s drawings would have exceeded 1/3 of its RCF, which is a trigger level for a significant margin increase on all its RCF drawings. As Kelly remarked, “Last year we saved in excess of £2m in interest in this way, so the system more than pays for itself.”
Reaping the rewards
Furthermore, Kelly said that the quality of conversation the treasury now has with the operating companies about forecasting is much improved. “Previously they were spending most of their time producing a basic model,” he recalled. “Now the system generates a basic model and we are able to get insights from the local teams, which allow us to better understand their spending plans, sales promotions, new contract wins and other areas which might affect our forecast – and which no one really had time to include before, unless they were very large.”
In summary, Kelly said, “The process of improving a group-wide cash forecast at an entity level is a little like painting the Forth bridge – a process which requires constant attention. For highly predictable cash flows such as payroll, treasury payments and many supplier payments, the forecast has close to 100% accuracy. The AI has also considerably reduced the number of significant unpredicted outflows. The reality is that the system allows us to analyse a lot more data and to a higher quality than we have ever been able to before.”
Discover more about how to improve your treasury core strength at International Treasury Management.